The Future of Data Management and Biostatistics: Trends and Technologies Shaping the Industry
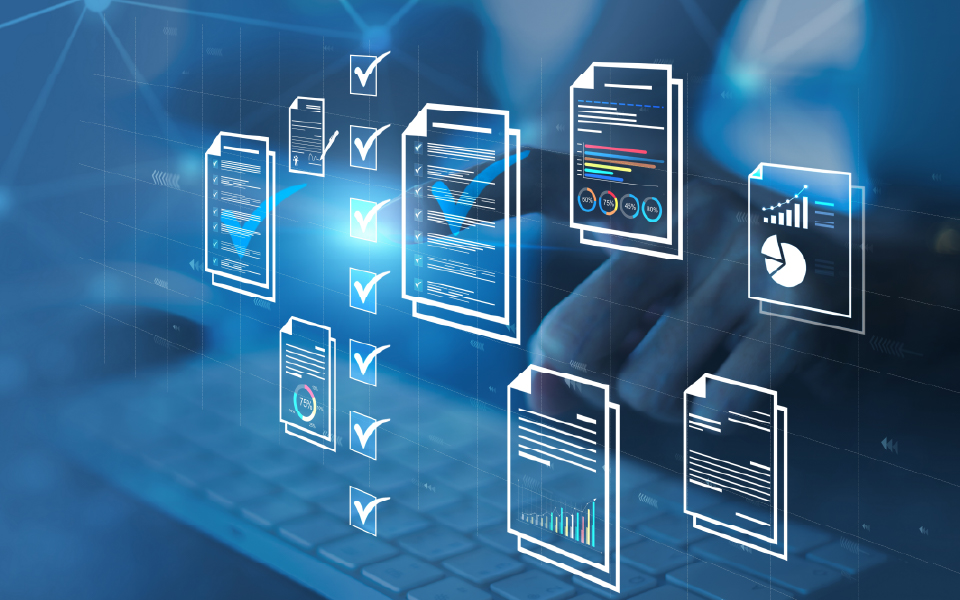
The integration of advanced technologies like artificial intelligence (AI) and machine learning (ML) is reshaping the fields of data management and biostatistics across drug development at a rapid pace. In fact, PwC recently shared that, “pharma companies that industrialize AI use cases completely across their organizations have the potential to double today’s operating profits by boosting revenues and reducing costs.
We expect that this industrialization process will begin to be fully realized by companies prioritizing AI by 2030.”
These technologies can play a pivotal role in automating processes, enhancing predictive modeling, and harnessing the power of big data. Let’s uncover how Sponsors of clinical trials can leverage these advancements to improve decision-making, enhance patient outcomes, and foster a more efficient clinical trial process.
Artificial Intelligence (AI) and Machine Learning (ML)
- AI and ML Integration: AI and ML are increasingly being integrated into data management and biostatistics to automate data cleaning, anomaly detection, and predictive analytics. These technologies can process vast amounts of data more quickly and accurately than traditional methods.
- Predictive Modeling: Machine learning models are being used to predict outcomes, optimize clinical trials, and identify patterns that might not be apparent through conventional statistical methods. By leveraging vast amounts of data, these models enhance decision-making processes and drive more effective strategies in clinical research.
Big Data and Real-World Evidence (RWE)
- Big Data Utilization: The explosion of big data, particularly from sources like electronic health records (EHRs), wearables, and social media, is providing new opportunities for biostatistics. Analyzing these large datasets requires sophisticated tools and techniques, like Datacise, to draw meaningful conclusions.
- RWE Integration: Real-world evidence (RWE) is becoming increasingly important in clinical decision-making and regulatory submissions. Data management systems are being developed to handle and analyze real-world data alongside traditional clinical trial data.
Cloud Computing and Data Warehousing
- Cloud Adoption: The adoption of cloud computing in data management is enabling more scalable and flexible data storage and processing solutions. Cloud platforms allow for real-time data sharing and collaboration across multiple locations.
- Data Warehousing: Advanced data warehousing solutions are being implemented to handle the growing volume and complexity of data. These warehouses support the integration of diverse data types, including structured and unstructured data.
Blockchain for Data Security and Integrity
- Blockchain Implementation: Blockchain technology is being explored for enhancing data security, ensuring data integrity, and maintaining transparent audit trails in clinical trials and other healthcare-related data management activities.
Regulatory Compliance and Data Privacy
- Enhanced Regulations: With the increasing complexity of data management, there is a growing focus on regulatory compliance, particularly around data privacy (e.g., GDPR and HIPAA). New technologies are being developed to help organizations manage compliance more effectively.
- Data Anonymization: Techniques for anonymizing data are becoming more sophisticated to ensure privacy while still allowing for valuable analysis of clinical data.
Interoperability and Standardization
- Data Standardization: There is a push towards standardizing data formats and terminologies (e.g., CDISC standards) to facilitate data sharing and integration across platforms and studies.
- Interoperability: Ensuring that different systems and technologies can work together seamlessly is a priority, driving the development of interoperable solutions in data management.
Patient-Centric Approaches
- Patient-Reported Outcomes (PROs): The inclusion of patient-reported outcomes is becoming more prevalent, requiring new tools for collecting and analyzing this type of data.
- Decentralized Trials: The shift towards decentralized clinical trials, which use digital tools to collect data remotely, is changing the landscape of data management and biostatistics, requiring new approaches to data collection, management, and analysis.
Data Visualization and Reporting
- Enhanced Visualization Tools: Advanced data visualization tools are being developed to help stakeholders better understand complex data through intuitive dashboards and interactive reports.
- Automated Reporting: Automation in reporting is becoming more sophisticated, reducing the time and effort required to generate regulatory submissions and other critical documents.
Collaboration and Open Science
- Open Science Initiatives: There is a growing trend towards open science, where data and research findings are shared openly with the scientific community, driving collaborative research efforts and accelerating the pace of discovery.
- Collaborative Platforms: Platforms that facilitate collaboration between researchers, data scientists, and biostatisticians are becoming more prevalent, allowing for more integrated and interdisciplinary approaches to data analysis.
The future of data management and biostatistics is set to be dynamic and transformative, driven by technological advancements and evolving industry needs. Organizations that embrace these trends and technologies will be better positioned to leverage data for improved decision-making, enhanced patient outcomes, and more efficient clinical research.
This article was written by MMS Senior Data Team Lead Olga Van Niekerk, based in Bloemfontein, South Africa.