Data Provenance in Real World Evidence Studies, Explained!
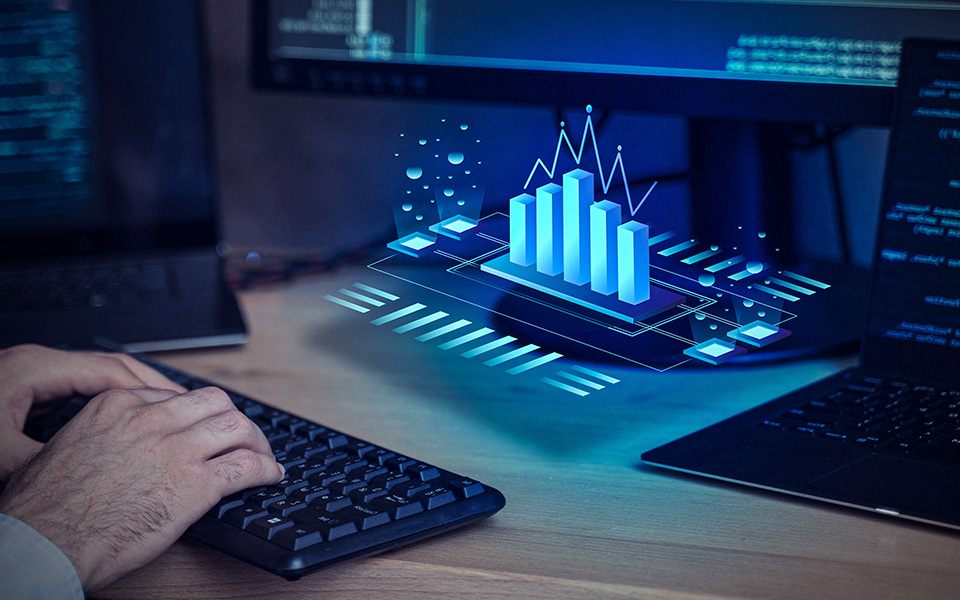
Data provenance in Real World Evidence (RWE) studies has quickly becoming an increasing focus in the industry, especially as 90% of pharmaceutical companies today have Real World Evidence teams according to Deloitte.
If an audit is underway, for instance, consider an auditor looking at the Real World Evidence (RWE) results and asking where the data point came from. Now, depending on whether the data provenance is set up within the data curation and an analysis processing stream, that question could become either simple or difficult to answer.
So, what is data provenance in Real World Evidence (RWE) studies?
Data provenance in Real World Evidence (RWE) studies is a way to “fingerprint” data at the source, allowing for it to be traceable through curation, transformation, and analysis steps. This way, when looking at the underlying detail of a visualization, or Tables, Listings, and Figures (TLFs), the original source can be found.
The Encyclopedia of Database Systems defines data provenance as:
“…a record trail that accounts for the origin of a piece of data (in a database, document or repository) together with an explanation of how and why it got to the present place.”
The key here is being able to trace all the way from the details providing summarized analysis results back to the original captured data. Ideally, data providence should play a role in the data visualization tool, such as Datacise® Explore.
Data provenance is becoming more important as a clinical study’s data volumes grow.
One billion rows of data!
With traditional clinical studies the amount of data collected and managed is relatively small compared to what is seen when working on Real World Data (RWD) for RWE studies. In cases like this, the data jumps several orders of magnitude in size, and it is common to work with more than 1,000,000,000 rows of data.
Given this enormity, the task of tracking is vital while moving through data curation and into a final place for analysis and visualization. And, since data provenance in Real World Evidence (RWE) studies is all about tracing data, it should be considered up front when designing the clinical study protocol.
The draft FDA guidance Real-World Data: Assessing Electronic Health Records and Medical Claims Data To Support Regulatory Decision-Making for Drug and Biological Products recommends the following:
“The study protocol and analysis plan should specify the data provenance (curation and transformation procedures used throughout the data life cycle) and describe how these procedures could affect data integrity and the overall validity of the study.”
Putting data provenance into practice
Given provenance is gaining attention as a best practice throughout the pharmaceutical industry and within the FDA, what can you do to prepare your next RWE study? Here’s five ways below.
- Have a good transfer protocol: When working with providers, set up a good transfer protocol to keep things simple. Identify up front identifying factors for each claim or intake record to allow for tracking back to the data provider. Keep in mind provenance doesn’t stop with you, and an agreement should be in place to allow provenance to be traced back through to vendors and their raw data. Additionally, catalog all providers, keep track of them, and track the types of files they send.
- Give IDs: For each file received and as the curation process begins, “stamp” each row with a ProvenanceID to globally name each row.
- Chain them together: As data moves through data curation and data analysis steps, keep the ProvenanceID’s. You may have to “chain” them together, as sources are joined.
- Cross reference everything: Establish a cross reference of data sources and ProvenanceID’s. This way, no matter where it is referenced within the data lifecycle, any data scientist can confidently get back to its source.
- Analyze it: Develop analysis using the underlying details, allowing provenance to be accurately and efficiently traced back to the source.
For visual learners, refer to Figure 1 below to understand how this can work.
Figure 1: An example of data provenance in Real World Evidence (RWE) studies
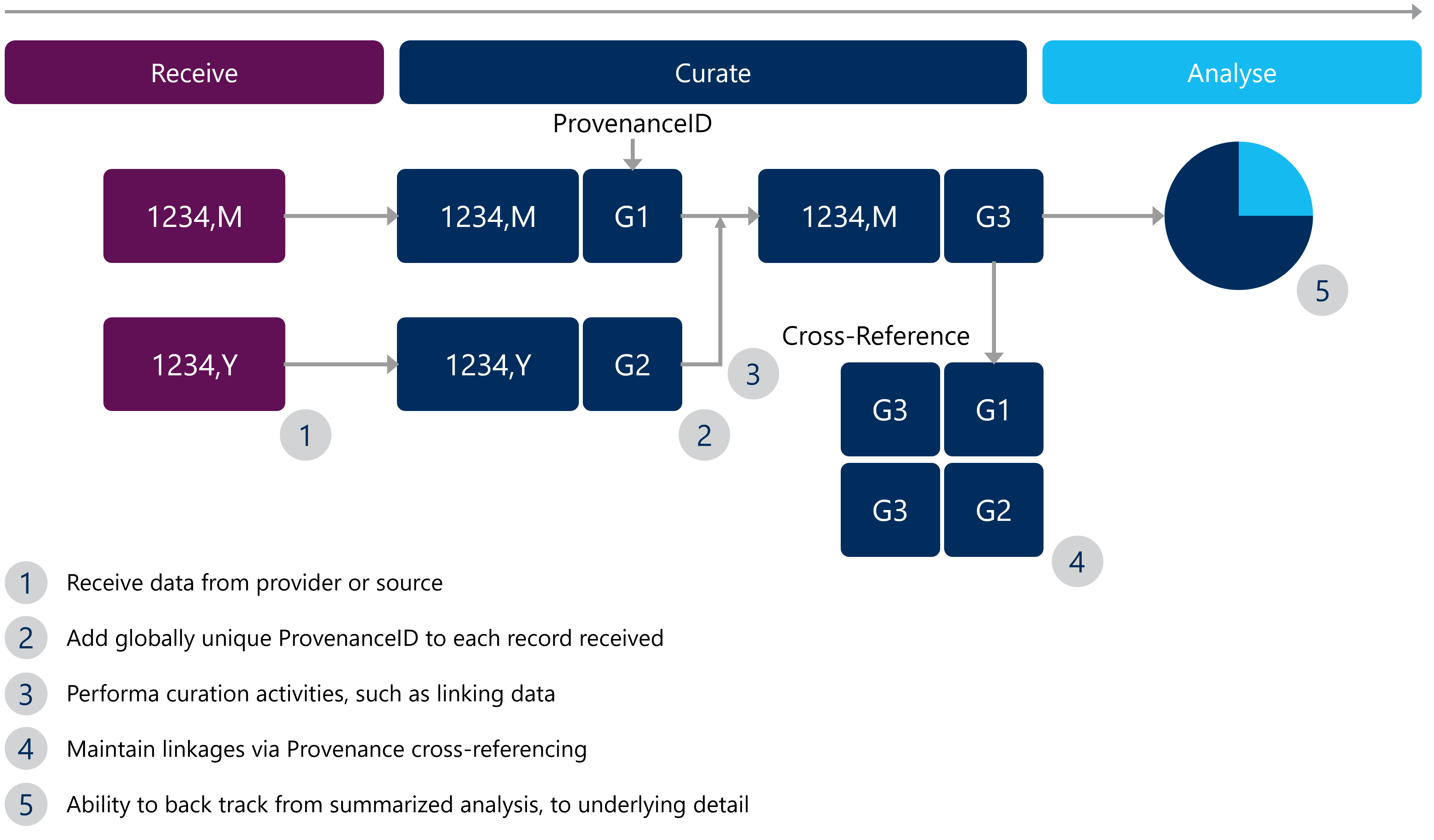
With data provenance in place and cross-referencing expanded, it is simple to see how this same scheme can be used to help understand data lineage, at least from a row point-of-view. Implementing the aforementioned data provenance tips can bring two key benefits to real world evidence (RWE) studies, including: checking data reliability and audit support.
Key Benefit: Checking Data Reliability
Once dashboards or other TLFs are compiled and going through review, someone may come to your data team regarding some outliers about their reliability. Questions may arise, including “Are they legitimate or are there data quality issues?”
When data provenance in Real World Evidence (RWE) studies is in place data scientists can trace back from the underlying details through the various transformation back to the source. By doing so, check-ins can occur along the way to compare the suspect data point to data at various points within the transformation and curation process.
Through this exercise, any data reliability issues, or lack thereof, will be evident.
Key Benefit: Audit Support
Data provenance in Real World Evidence (RWE) studies can be used to show GxP auditors the path that data takes through the curation process. Trace data from its source to visualization, or vice versa.
If ProvenanceID’s are created to be globally unique, and the correct cross referencing is set-up as seen in Datacise® Curate, it becomes easy to report on an item in either direction.
In both cases being comfortable generating an audit trail is important as pointed out in section III, C. 2. Audit Trail within the FDA’s Guidance for Industry Part 11, Electronic Records; Electronic Signatures — Scope and Application (Section III, C, 2.). Though the requirement to keep an audit trail is not always explicitly stated, one becomes important “to ensure trustworthiness and reliability of the records.”
Having a clear ways to identify source data and trace it though the data curation and transformation processes is essential. As US FDA draft guidelines propose, incorporating data provenance up-front when designing the clinical study protocol and data analysis plan is key.
Once data provenance is in place it becomes a great tool to help answer questions regarding data quality and strengthen any audits.
To explore how we can support your specific needs regarding data provenance in real world evidence studies, please click here to start a conversation with our experts today.
Authored by: Kris Wenzel, Senior Manager, Data Science