Clinical Data Science: Five Ways it Evolved from Clinical Data Management
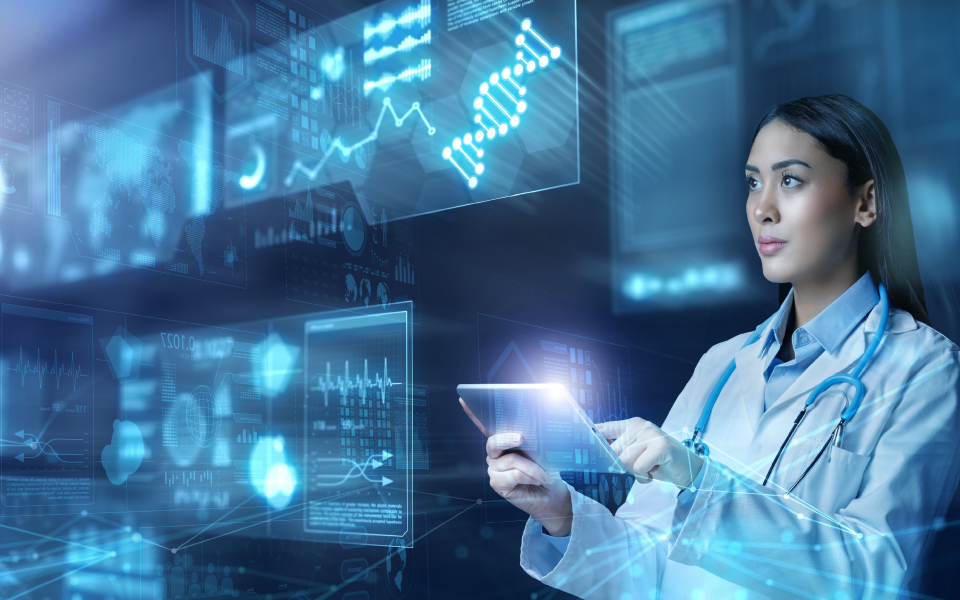
Clinical data science is growing exponentially, as the Bureau of Labor Statistics confirms that the need for data scientists is rising faster than other areas with a 35 percent project growth rate through 2032. And, as the pharmaceutical industry has seen in the last decade, clinical research industry has also grown and evolved in many aspects.
Clinical trial data is growing exponentially, trials are now more global, datasets are more diverse, and data generated during the trials are massive. With that, clinical trials have begun qualifying as complex and big data.
Big data means high in volume, high velocity, high veracity, and high variety.
As there is a shift from the traditional clinical data management practices to more advanced analytical and data-driven approaches, the pharmaceutical industry is experiencing greater evolution due to the rapid increase in digitalization of healthcare data, and the evidence-based approach in decision-making in clinical practice.
What’s the difference?
To understand how clinical data science has evolved from clinical data management, a proper definition must first be set.
Clinical Data Management is a process of collecting, organizing, managing, and analysing the clinical trial data in compliance with regulatory guidelines. Data accuracy and completeness are of utmost importance. This process is necessary to ensure the validity and reliability of the clinical data while preserving its accuracy and integrity.
Clinical Data Science is the strategic discipline that empowers data-driven approaches in clinical research, ensuring subject protection and the reliability of trial results. It involves processes, domain expertise, technologies, data analytics, and adherence to Good Clinical Data Management Practices, all essential for informed decision-making throughout the clinical research life cycle.
As the industry has progressed, clinical data science has emerged from the clinical data management function to stand on its own, and the following five areas detail this evolution.
Data collection and integration
Systems used for clinical data science have adopted more advanced databases, integrating data from various sources to enable real-time data analysis and decision-making. This integration has allowed to identify the patterns, trends, and anomalies in the data.
One such system is Datacise, which allows Sponsors to immediately analyze and visualize clinical data, Real-World Data (RWD), and data from other data sources.
Advanced analytics and machine learning
Machine learning and artificial intelligence (AI) algorithms play a crucial role in clinical data science. These technologies help in converting the data for analysis into a structured format. Analyse large datasets, associations, prediction of outcomes and support clinical decision-making. These tools can assist in automation of data management processes, like data cleaning, data validation, and data integration.
Real-time data analysis and monitoring
Traditional clinical data management focused on site monitoring, retrospective analysis like post-trial data analysis, and regulatory reporting. With the shift and high demand towards real-time data analysis and monitoring, expenditures of time and money can greatly be reduced for an organization. Clinical data science enables clinicians to extract real-time insights to data, monitor patient progress, detect any anomalies, and make timely and accurate interventions to improve patient outcomes.
Integration of clinical research and practice
Clinical data science aims to bridge the gap between clinical research findings and application in clinical practice, which in turn improves patient care and outcomes. Research can conduct observational studies, working on real-world evidence, generate evidence for treatment guidelines which is done by leveraging large-scale clinical datasets. Integration of research and practice evaluates the effectiveness of interventions in real-world settings. This promotes evidence-based medicine and improves patient care.
Ethical consideration and data governance
As there is a continuous increase in patient data to ensure privacy, data security and ethical data use becomes critical. Data governance framework are being developed to protect patient privacy, maintain data confidentiality, and ensure compliance with pharmaceutical industry regulations, like GDPR.
The future of clinical data science is further expanded when exploring the integration of genomics and personalized medicine. With the advent genomic sequencing technologies, researchers can now analyse an individual’s genetic makeup and identify specific genetic markers associated with diseases or drug responses lead to personalized approaches or tailored treatment plans. This can help in finding more effective and targeted therapies.
Thus, emergence of big data analytics and data mining techniques signifies a shift towards more advanced analysis techniques, integrations of genomics, and digital technologies has transformed clinical data management in clinical data science.
To speak with a clinical data science expert, please click here and we will connect you with an expert.
Authored by:
Anjana Muralidhar, Manager, Clinical Data Management.