Partner Spotlight: A Conversation with Julia Computing
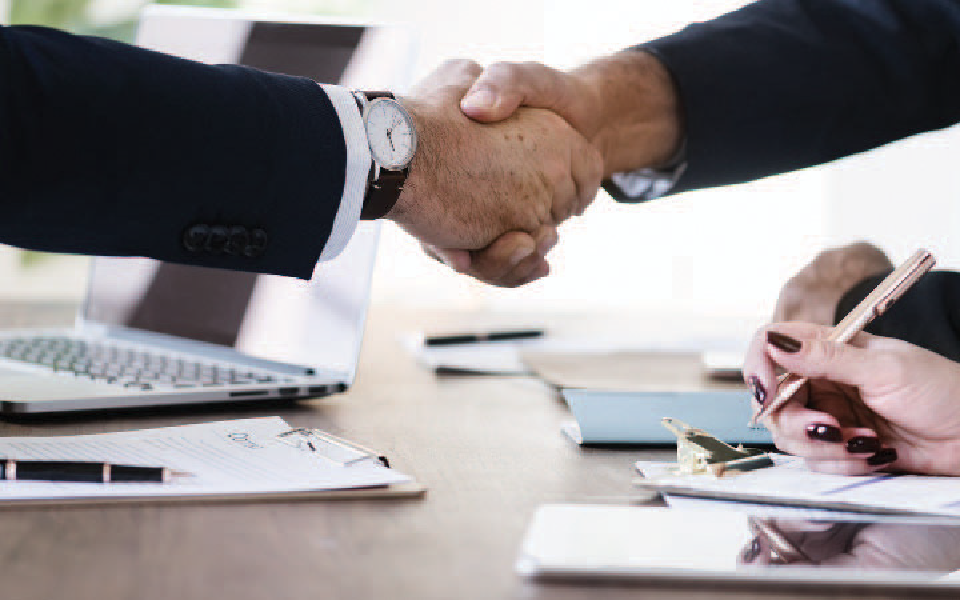
As an integral member of the Health Analytics Collective, Julia Computing is seen as the programming language of the future. We sat down with Andrew Claster, Director of Marketing & Communication at Julia Computing to get a sense of what Julia is and where they are headed.
How did Julia Computing come to be in a day and age where there are so many languages already?
In 2012, the conventional wisdom in computer science was that a programming language could be fast or productive, but not both. Julia co-creators Jeff Bezanson, Stefan Karpinski, Viral Shah and Alan Edelman questioned this premise. They set out to create a new language with all the best features and none of the negative attributes of C, Ruby, Lisp, Python, R and Perl. As Jeff, Stefan, Viral and Alan explained in their Feb 14, 2012 blog post “Why We Created Julia”:
In short, because we are greedy.
We are power Matlab users. Some of us are Lisp hackers. Some are Pythonistas, others Rubyists, still others Perl hackers. There are those of us who used Mathematica before we could grow facial hair. There are those who still can’t grow facial hair. We’ve generated more R plots than any sane person should. C is our desert island programming language.
We love all of these languages; they are wonderful and powerful. For the work we do — scientific computing, machine learning, data mining, large-scale linear algebra, distributed and parallel computing — each one is perfect for some aspects of the work and terrible for others. Each one is a trade-off.
We are greedy: we want more.
We want a language that’s open source, with a liberal license. We want the speed of C with the dynamism of Ruby. We want a language that’s homoiconic, with true macros like Lisp, but with obvious, familiar mathematical notation like Matlab. We want something as usable for general programming as Python, as easy for statistics as R, as natural for string processing as Perl, as powerful for linear algebra as Matlab, as good at gluing programs together as the shell. Something that is dirt simple to learn, yet keeps the most serious hackers happy. We want it interactive and we want it compiled.
(Did we mention it should be as fast as C?)
While we’re being demanding, we want something that provides the distributed power of Hadoop — without the kilobytes of boilerplate Java and XML; without being forced to sift through gigabytes of log files on hundreds of machines to find our bugs. We want the power without the layers of impenetrable complexity. We want to write simple scalar loops that compile down to tight machine code using just the registers on a single CPU. We want to write A*B and launch a thousand computations on a thousand machines, calculating a vast matrix product together.
We never want to mention types when we don’t feel like it. But when we need polymorphic functions, we want to use generic programming to write an algorithm just once and apply it to an infinite lattice of types; we want to use multiple dispatch to efficiently pick the best method for all of a function’s arguments, from dozens of method definitions, providing common functionality across drastically different types. Despite all this power, we want the language to be simple and clean.
All this doesn’t seem like too much to ask for, does it?
How can Julia Computing add value to the mission of the HAC?
According to MIT News, the Health Analytics Collective seeks to “significantly shorten the approval process for pharmaceuticals.”
Julia is more efficient for big data. Julia was used to analyze and catalog 188 million stars, galaxies, and other astronomical objects in just 14.6 minutes on one of the world’s five fastest supercomputers. This means that Julia can analyze voluminous patient data, such as second-by-second heart rate or blood pressure readings, for thousands or millions of patients in a very short amount of time.
What are the major challenges with Julia across the industries where it is being used?
One of the biggest challenges with Julia is legacy software. Some firms haven’t yet made the leap to Julia because they have a lot of legacy code in Python or other languages. Julia has been downloaded more than 8.4 million times and has more than 2,500 packages. It is interoperable with C, Java, Python, R and other languages, but some users prefer to stick to a slower language they know rather than a faster language they have never used before. And while 8.4 million Julia downloads means there are a lot of Julia programmers out there, it can be challenging for some companies to find Julia programmers who have the industry-specific knowledge and experience that they require.
Is Julia the “go to” for Machine learning and AI?
According to some of Facebook’s top artificial intelligence executives, Chief Scientist and Turing Award Winner Yann LeCun said that “Deep learning may need a new programming language,” emphasizing that “Julia and Swift are nice.” Facebook Artificial Intelligence Researcher Soumith Chintala added that “A lot of folks ask me: what’s the next mainstream ML software? What is PyTorch-next? There is no magic answer, it depends on where the field goes … If GraphConvNets, then Julia — for its ability to build efficient fundamental data structures in an interactive language.”
Additionally, Google Brain’s artificial intelligence team says: “Julia is another great language with an open and active community. They are currently investing in machine learning techniques, and even have good interoperability with Python APIs. The Julia community shares many common values as with our project, which they published in a very like-minded blog post after our project was well underway. We are not experts in Julia, but since its compilation approach is based on type specialization, it may have enough of a representation and infrastructure to host the Graph Program Extraction techniques we rely on.”
Where do you see Julia in the next decade?
In addition to our work with the Health Analytics Collective, we hope Julia will continue to drive artificial intelligence and machine learning to new heights in fields as diverse as RNA sequencing, mapping global genetic diversity, modeling cancer evolution, precision medicine, surgery using augmented reality, medical diagnosis, analyzing the migration of genes, animals, plants and humans in response to climate change, robotics, self-driving cars, cryptography, astronomy and agriculture.